Rahul Gupta: "Federated learning for industrial systems"
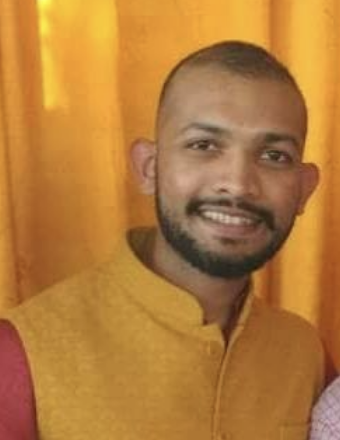
Title: Federated learning for industrial systems
Abstract: Machine Learning (ML) systems are getting increasingly popular, and drive a number of applications and services in our daily life. Given the increasing prominence of ML driven systems, Federated Learning offers a way to train models in a secure manner without requiring access to private data. This is attractive for industrial model training as this allows model training at scale without mandating data donation from end users of the technology. In this talk, we will present a summary of industrially scaling federated learning and discuss (i) considerations for applying federated learning to real world (e.g. distribution of data across “heavy users” versus “light users”) (ii) conducting Federated Learning with labels generated using noisy feedback from end users of the technology and, (iii) training models with data obtained from mixed distributions (with applications to neural machine translation). We hope to gain audience’s attention on scaling federated learning to industrial applications and foster research given the challenges.
Bio: Rahul Gupta is an Applied Science manager at the Spoken Language Understanding Innovations (SLU-Innovations) team in Cambridge, Massachusetts. Since joining the Alexa organization, he has focused on designing NLU models for scalability and speed. Some of his more recent research focuses on Trustworthy Machine Learning with emphasis on privacy preserving techniques, fairness and federated learning. He received his PhD from the University of Southern California in 2016 on interpreting non-verbal communications in human interaction. He has published over seventy papers in avenues such as EMNLP, ACL, NAACL, ACM Facct, IEEE-Transactions of affective computing, IEEE-Spoken language Understanding workshop, ICASSP, Interspeech and Elselvier computer speech and language journal. He is also co-inventor on ten patent pending technologies at Amazon.